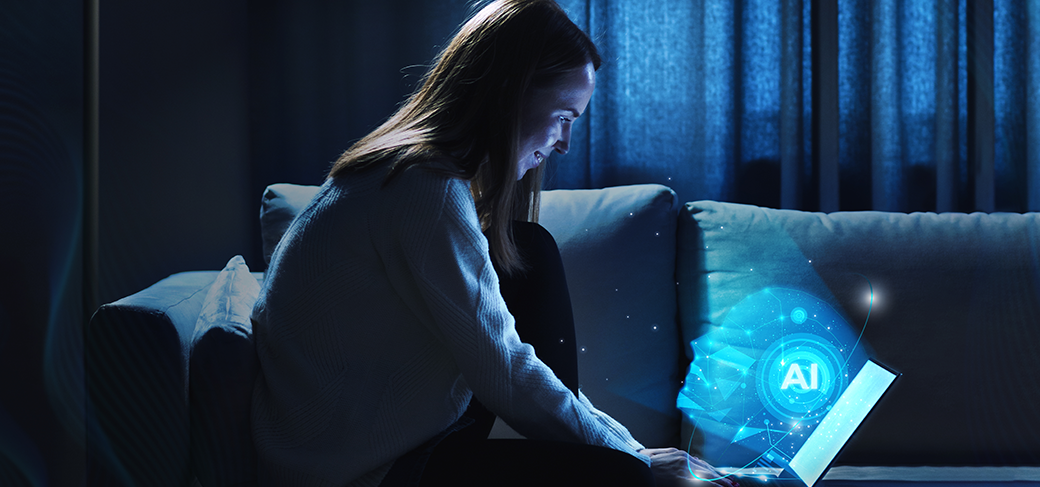
Revolutionizing Retail: How Agentic AI is Transforming Demand Forecasting, Inventory Management, and Dynamic Pricing
Revolutionizing Retail: How Agentic AI is Transforming Demand Forecasting, Inventory Management, and Dynamic Pricing
The retail industry is at the forefront of this technological evolution, with Agentic AI in the driver's seat for optimizing various business processes. In response to the increasing need for a streamlining of customer experiences, retailers are willingly turning to autonomous AI systems for sufficiently managed operations.
In this blog, we will deeply analyze how Agentic AI is transforming three areas that are critical to retail:
1. Demand forecasting
2. Inventory management
3. Dynamic pricing
Through advanced algorithms and intelligent systems, retailers can now achieve optimal stock levels, reduce human errors, and enhance overall operational efficiency.
What is Agentic AI in Retail?
Agentic AI refers to autonomous artificial intelligence systems that can make decisions and take actions independently of human intervention. In the retail Industry, Agentic AI is used to enhance various business operations, including demand forecasting, inventory management, dynamic pricing, and customer service. Agentic AI agents are capable of going through tremendous data loads, looking for patterns, predicting outcomes, and performing tasks in real time. This degree of autonomy allows retailers to automate manual processes, optimize the whole operation, and rapidly respond to market changes.
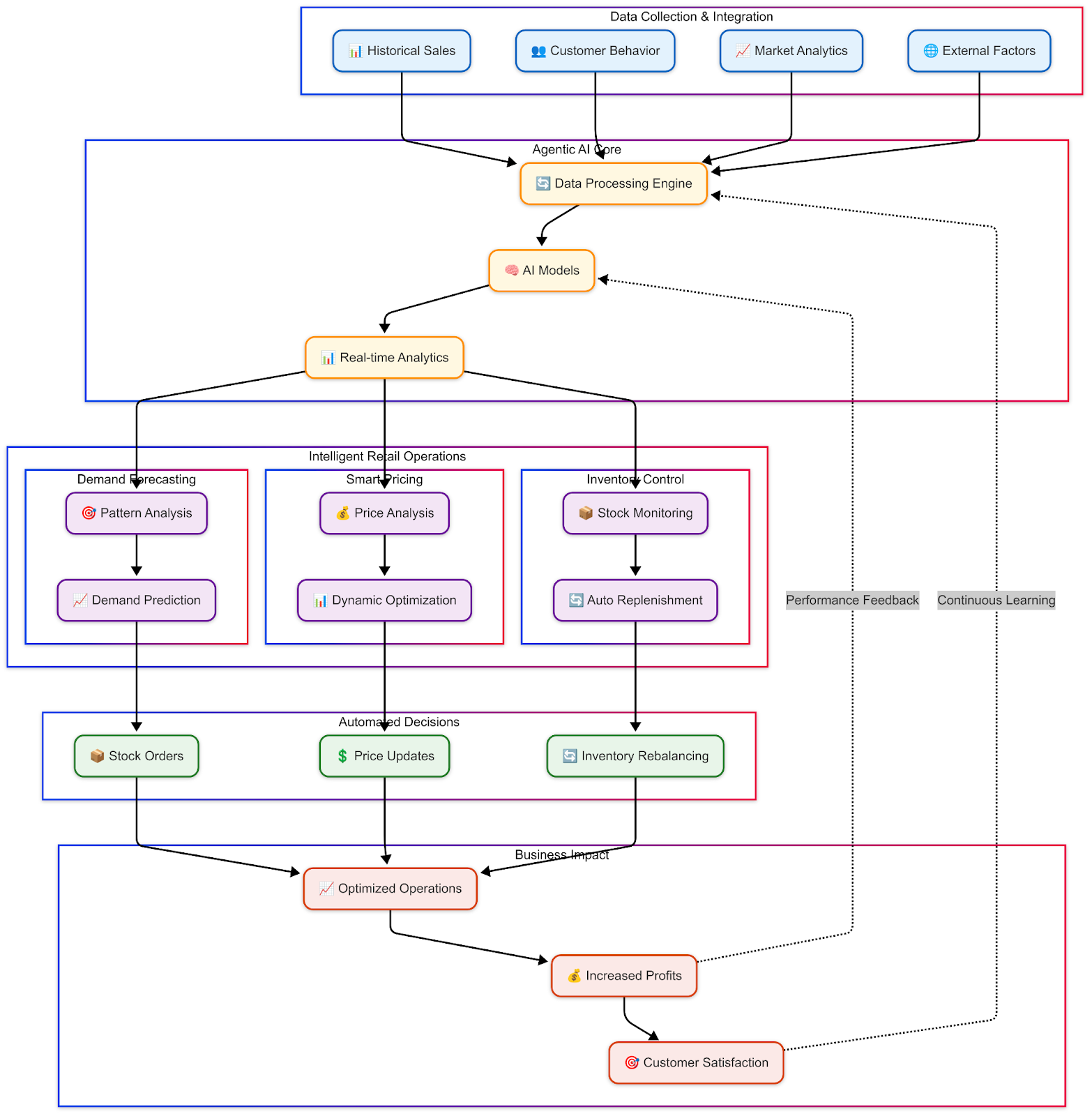
1. Demand Forecasting: Predicting the Future of Retail
Effective demand forecasting is the backbone of inventory management. Without credible forecasts, retailers are bound to overstock merchandise and incur excess storage and carrying costs or understock merchandise, resulting in stockouts and lost sales. While traditional demand forecasting methods have centered around manual methods and simple statistical models, Agentic AI is taking forecasting to the next level.
The Problem: Meet the Accurate Demand
Retailers often struggle to predict customer demand due to multiple variables such as seasonality, changing customer preferences, economic shifts, and even external factors like weather. Traditional forecasting methods cannot always capture the complexities of these variables, leading to forecasting errors and inefficient stock levels.
The Solution: AI-Driven Demand Forecasting
Agentic AI makes demand forecasting an intelligence-driven, data-rich process employing Machine Learning (ML). With the use of AI, permutations of data from historical sales to consumer behaviors can be analyzed, thus predicting future demand accurately.
Here is how Agentic AI does demand forecasting:
Data Integration: AI systems assemble data from various sources: historical sales data, customer purchasing behavior, supply chain modeling, and externalities like market trends and promotions. This data is updated through real-time loading.
Machine Learning Models: Time Series Analysis (ARIMA, LSTM) and Regression Models are used to find general patterns in past demand. RL algorithms allow the system to continuously learn and improve predictions based on open-loop feedback from the real world.
Advanced Accuracy: Agentic AI thereby combines the predictions of several models in an ensemble fashion, which includes factors pertaining to external happenings and market dynamics.
Future demand predicts that retailers will plan in advance, avoiding any mismatch, both in stock-outs and over-stocking.
2. Inventory management: Automation with AI agents
One of the following key steps in inventory management after demand forecasting is making an effort toward it. In doing this, Agentic AI comes in to automate and optimize stock levels by reducing human fingers and stock management errors.
The Problem: Inefficient and Error-Prone Inventory Management
Traditional inventory management methods are traditionally time-consuming manual processes, including stock review, stock planning, and communication with Vendors. These processes are time-consuming, prone to errors, and often lack the flexibility to respond to real-time changes in demand or supply. This might make it hard for retailers to manage their inventories, prompting either overstocking (higher holding costs) or stockouts (lost revenues).
The Solution: Autonomous Inventory Management
Agentic AI modernizes inventory management by autonomous AI agents making real-time, data-driven decisions. The main AI analyses data from various sources: sales, stock level, and supply-chain information components, among others, to ensure the availability of the right product in good quantity at the right time.
Here’s how Agentic AI optimizes inventory management:
Stock Replenishment: Once demand is forecasted, AI agents ensure timely stock replenishment by calculating optimal order quantities and timing. These decisions are based on real-time stock levels, lead times, and supplier performance.
a. Calculation of Safety Stock: AI calculates buffer stock levels that help to prevent stockouts by handling demand variability and supplier lead times.
b. JIT replenishment: The agent calculates the best time to reorder a product to ensure that stock levels are maintained without piling too much.
Autonomous Ordering: When stock levels reach a predefined threshold, AI agents autonomously reorder products. They can handle supplier communication, initiate orders, and even reroute orders to alternative suppliers if necessary.
Stockout Prevention: AI systems monitor inventory in real time, predicting potential stockouts based on sales velocity, supply chain delays, or demand spikes. When stock is running low, AI triggers reorders, ensuring stock is replenished before it runs out.
Dynamic Adjustments: In case of sudden demand changes (e.g., due to promotions or external factors like holidays), AI agents adjust stock orders dynamically to meet the new demand.
Using optimization algorithms and linear programming remains a way in which Agentic AI continuously tunes its replenishment, minimizing inventory costs and ensuring product availability.
3. Dynamic Pricing: Optimizing Profitability and Competitiveness with AI
Dynamic pricing is one area in which Agentic AI is having its most significant impact. The nature of today's fast-paced retail environment dictates that, prices must evaluate in real-time changes based on external factors, including customer demand, competitor pricing, seasonal trends, and stock availability. Traditional pricing processes are rigid in that they often do not allow a responsive price for market changes, but Agentic AI would permit the retailer to apply high-end dynamic pricing strategies that maximize profit margin and combat competitiveness.
The Problem: Pricing Optimization in a Dynamic Market
In competitive markets, retailers often find themselves challenged with pricing. Underpriced merchandise means lost revenues; overpriced goods mean dissatisfied customers or lost market share. The dilemma revolves around securing profitability against competitive pricing whilst keeping in mind customer expectations.
The Solution: AI-Powered Dynamic Pricing
Agentic AI offers dynamic pricing that analyzes tremendous volumes of real-time data to trigger any adjustments in pricing accordingly. The AI agents analyze data such as demand for the product, competitor prices, available stock, and external market conditions; all these factors influence the determining pricing through some advanced machine learning algorithms.
Here is how Agentic AI accomplishes the task of dynamic pricing:
Real-Time Intelligence Upon Demand: In executing pricing, AI agents collect data on customer behavior, competitor pricing, and stock levels to inform pricing decisions.
Price Elasticity Prediction: AI systems, through machine learning, inform about how sensitive the demand is to changes in price (price elasticity). It equips retailers with data on setting prices that will maximize either sales volume or profit.
Stock Level: AI adjusts prices of products according to stock availability. In the case of limited stock, the AI price goes up as the parameter to maximize profitability out of such scarcity.
Competitor Price Monitoring: The AI continuously tracks competitor pricing and adjusts prices to remain competitive without sacrificing margins. If a competitor lowers their price, the AI may reduce the price slightly to capture market share.
Seasonal Adjustment: AI adjusts prices according to seasonal trends, for instance, giving out discounts during the off-season or boosting them during high seasons like holidays or special events.
For instance, imagine an e-commerce platform selling electronics. If a popular smartphone model experiences high demand, the AI agent will dynamically increase the price to capture the higher willingness to pay. Conversely, if stock levels are high but demand is low, the AI may lower prices to encourage sales and clear excess inventory.
Agentic AI has the potential to transform the retail industry through a wide array of advanced technologies.
- Machine Learning is used for applications like demand forecasting, predictive analysis, and dynamic pricing -optimization.
- Natural Language Processing allows store managers to interact with the system using voice or text queries.
- Optimization algorithms are used for stock replenishment strategies, cost minimization, and dynamic price changes.
- Reinforcement learning allows for adjustments to be made dynamically, as well as for continued learning based on real-time feedback.
- Cloud Computing lends itself to real-time data integration and scalability and ensures seamless systems operation across various store locations.
The Benefits of Agentic AI in Demand Forecasting, Inventory Management, and Dynamic Pricing
With Agentic AI, retailers can gain a number of benefits. Some of these include:
1. Reduction of Human Error: With automation, the chances of any human errors related to stock level, ordering, demand forecasting, and pricing are minimized.
2. Enhanced Efficiency: AI agents take away repetitive and tedious tasks, empowering human resources to do higher-level work.
3. Cost Optimization: Good forecasting of demand, optimized inventory levels, and dynamic pricing strategies are capable of reducing carrying costs, stock-outs, and missed revenue opportunities.
4. Real-Time Adaptivity: AI keeps learning and assimilating all the time, ensuring that inventory strategies and pricing stay relevant in an ever-changing retail world.
5. Scalable: AI systems are able to scale to support a multitude of large inventories and dynamic pricing strategies across many stores or online retailers as needed.
Conclusion: The Future of Retail with Agentic AI
Agentic AI is going to redefine retail, improving efficiency, reliability, and adaptability. Warehouse automation by AI-driven demand forecasting, autonomous inventory management, and dynamic pricing allows for lower stock levels, increased profitability, and customer satisfaction. The ongoing development of this technology is open to the complete autonomy of a retail operation, thus laying the foundation for smarter, responsive retail ecosystems.
By embracing Agentic AI, retailers can unlock a future where inventory is always aligned with customer demand, prices reflect real-time market conditions, and operations run seamlessly to meet the ever-growing expectations of the retail world. In a competitive landscape, this technological innovation is not just a luxury—it’s a necessity for staying ahead.